An automation is only as good as the data you feed into it.
If you build an automation on bad data, you’ll get a messy automation that’s prone to errors and bugs. If you use high-quality data, you can create a useful and reliable automated workflow.
While you might be eager to start automating your company’s workflows right away, it’s best to ensure that your data is ready for automation before you begin.
In this post, we’ll show you the difference between good data and bad data, and we’ll explain how using the right data will prevent all sorts of problems.
Avoid redundancies in your data
Good data doesn’t have any redundancies
You don’t want to have multiple records for the same person, or the same company, or the same project that you’re tracking.
Redundancy makes it hard to use your data in any capacity, but it’s especially frustrating when you’re trying to build automations.
With a redundant dataset, you might end up running an onboarding automation ten times for the same client, or setting the same task repeatedly for a given project.
If you want to use a dataset for automation, make sure that you only have one entry for each item that you want to track.
Only track relevant attributes
Good data has relevant attributes - and only relevant attributes.
When you’re creating records, it can be tempting to make a field for every conceivable attribute.
But if you’re looking to build automations with your datasets, you should limit your databases to only include fields that are relevant for whatever you’re tracking.
If you’re maintaining a database of the clients you work with, you’ll probably want to include things like their company name, your main point of contact at the company, or their start date - in other words, information that’s relevant to your relationship and the automation you want to build.
In general, you should only include attributes that you intend to use or track. Otherwise, you’ll end up with a cluttered, messy database.
Irrelevant attributes can make it harder to build automations as you comb through a bunch of useless data to find what you’re looking for.
It can also make it harder to add complete records to the database, as all of the superfluous fields encourage your team to take shortcuts and post incomplete records.
Make sure your records are complete
While we’re on the subject, Good data is complete!
Whatever attributes you choose to add to your database, make sure that they’re filled out completely for every record.
If you build an automation connected to an incomplete database, it could prevent the automation from working properly.
For example, let’s say one of your automations is supposed to send out reminders to your client an hour before each of your meetings.
If the client’s “email” field is blank, the automation won’t be able to send them anything, and will just return an error.
Keeping complete records is always a good practice, but it’s especially important if you’re building automations that rely on those records.
Build datasets that easily reveal key insights
Good data can be summarized to easily find key takeaways.
You should be able to take a quick look at your database and immediately see some of your most important metrics.
Your “Projects” database should be able to show you at a glance how many projects your team is working on right now, and which projects have outstanding work or are still awaiting payment.
Getting good summaries out of your data will often depend on many of the other things we’ve talked about in this video.
If you have complete, consistent records with no redundancies, it should be pretty straightforward to find the takeaways that you need.
If you’re not able to learn what you need to at a glance, then you’ll probably need to go back and rethink what attributes you’re tracking.
Once you’re collecting the right datapoints, you’ll easily get the insights you’re looking for.
It all starts with the data
No-code automation is one of the best strategies for optimizing your business and saving time. But before you start connecting your apps in Zapier, Make, or Unito, you need to make sure the data you want to work with is ready to support automation.
Once you’re sure that your data has no redundancies, tracks relevant attributes, contains complete records, and shows you useful insights, you can start building time-saving automations that remove robotic tasks from your workflows forever.
If you’d like to learn more about no-code automation and managing your data, check out our blog or our YouTube channel. You can also follow XRay on Twitter, Facebook, or LinkedIn.
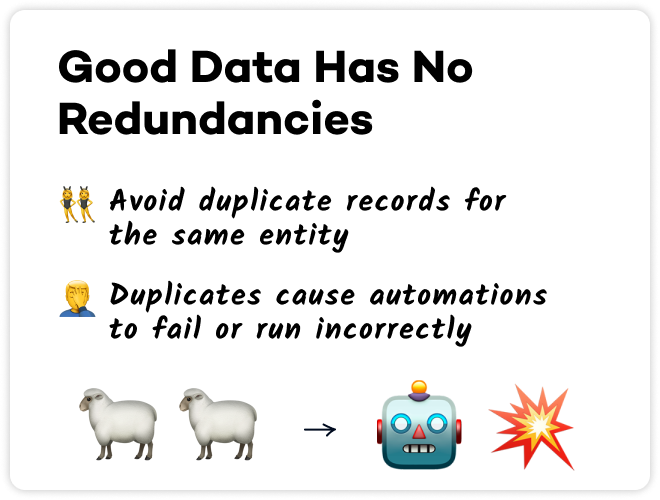
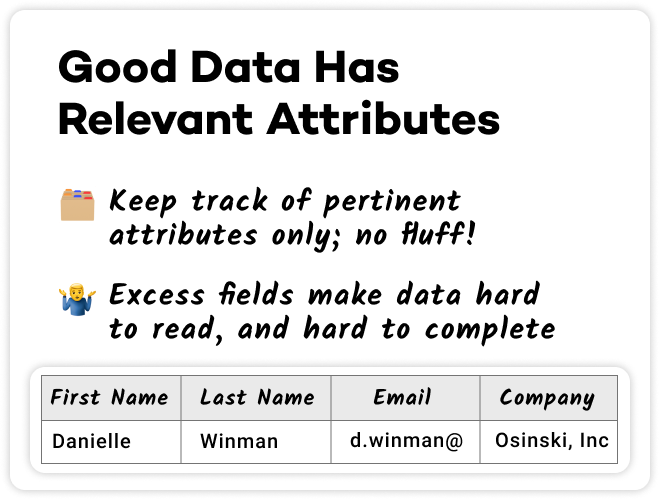
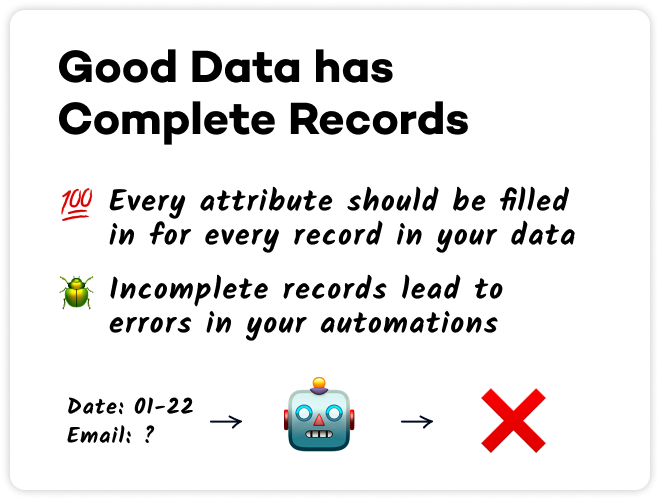
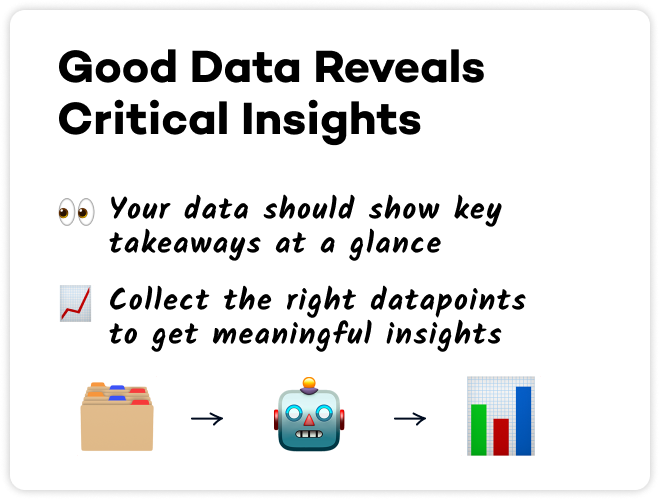